Max Hinne
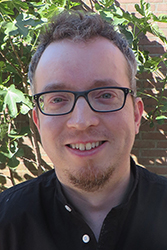
I am an assistant professor at the Department of Artificial Intelligence, at the Radboud University, Nijmegen, The Netherlands, leading the Uncertainty in Complex Systems group.
There are few objects more interesting than the brain. It has just the right structure and dynamics that results in large-scale information processing, from which our complex cognition emerges and using which it ultimately studies itself.
In my research, I use Bayesian modelling & statistics to study latent (network) structures in the human brain, via neuroimaging modalities such as fMRI and dwMRI, and to use these as predictive models. I am particularly interested in Bayesian nonparametric models (such as Gaussian processes), in which the complexity of the model is learned from data instead of provided a priori. Other, related, interests incude (quasi-)causal inference, predictive processing, and dynamical systems.
My contact information is listed below.
“All stable processes we shall predict. All unstable processes we shall control.”
John von Neumann
Master thesis projects
Interested in doing a master thesis on topics such as applying or developing new Bayesian nonparametric methods to neuroimaging data? Send me an email!
Publications
PhD thesis
On June 9th 2017, I defended my doctoral thesis entitled Bayesian Connectomics: a probabilistic perspective on brain networks with a cum laude distinction.
Publications
For an up-to-date overview of my publications, please visit my Google Scholar profile.
Software
BaCon
The Bayesian Connectomics Toolbox contains Matlab/MEX scripts that implement MCMC approximations for:
- Structural connectivity using dMRI probabilistic streamline count data, using either a prior on network density (struc_conn_density_prior.m) or on clustering (struc_conn_irm_prior.m).
- Functional connectivity using fMRI BOLD time courses data and a structural connectivity estimate (gwishrnd.m).
- Simultaneous estimation of structural and functional connectivity based on fMRI BOLD (ggm_gwish_cbf_direct.m).
Basic usage of each of the scripts is demonstrated in demo.m. Feel free to email me with any questions or comments.
Latent space modeling
Matlab code and Stan models are available for the latent space model that was used for link prediction. Running the sample requires that you have Stan and the MatlabStan interface installed.
GP CaKe
Code for the causal inference / effective connectivity estimation using GP CaKe is available here. There is a tutorial for GP CaKe in the form of a series of blog post, which you can read on the MindCodec website.
Contact information
Online
- m.hinne@donders.ru.nl
- http://nl.linkedin.com/in/mhinne
- Skype
- max.hinne
- Google Scholar
- Max Hinne
Offline
Nijmegen
- Phone
+31-24-3612554
- Visiting Address AI
Room MM 02.353
Thomas van Aquinostraat 4
6525 HR Nijmegen